Interpretable AI Agents
We work on methods to interpret or explain autonomous agents, with a focus on those that use artificial intelligence-based decision making policies.
This work was funded in part by ONR N00014-20-1-2249.
Searching for temporal logic explanations of reinforcement learning agents
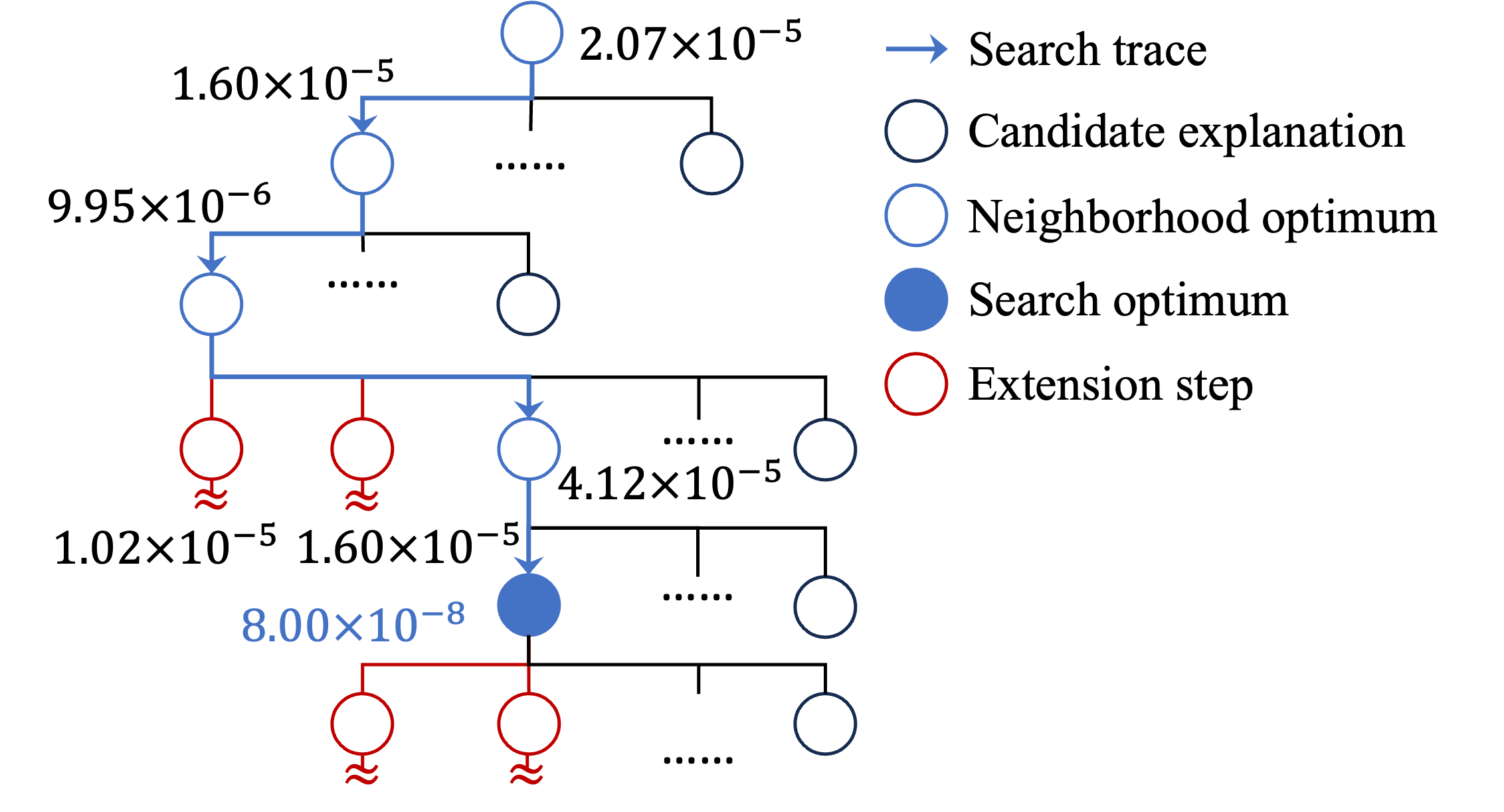
This work proposes a greedy search over a class of temporal logic formulae to infer human-interpretable explanations of reinforcement learning policies. See our IEEE Control Systems Letters paper for more details.