Intelligent Transportation Systems
We work on artificial intelligence and machine learning methods to improve autonomy and traffic management in intelligent transportation systems (ITS).
Learning emergent coordination in collective autonomous air mobility systems (CAMS)
This work explores reinforcement learning algorithms for encouraging emergent cooperation in autonomous air mobility vehicles, with consideration of heterogeneity (e.g., individual objectives) and the overall integrated system (e.g., vehicles and their interactiosn with operators and services).
Evaluating STGNNs for air transportation networks

This work builds upon our earlier work using STGNNs for railway networks by exploring potential benefits of STGNNs with various graph convolution operations towards delay prediction in air transportation networks.
Predicting delays in railway networks
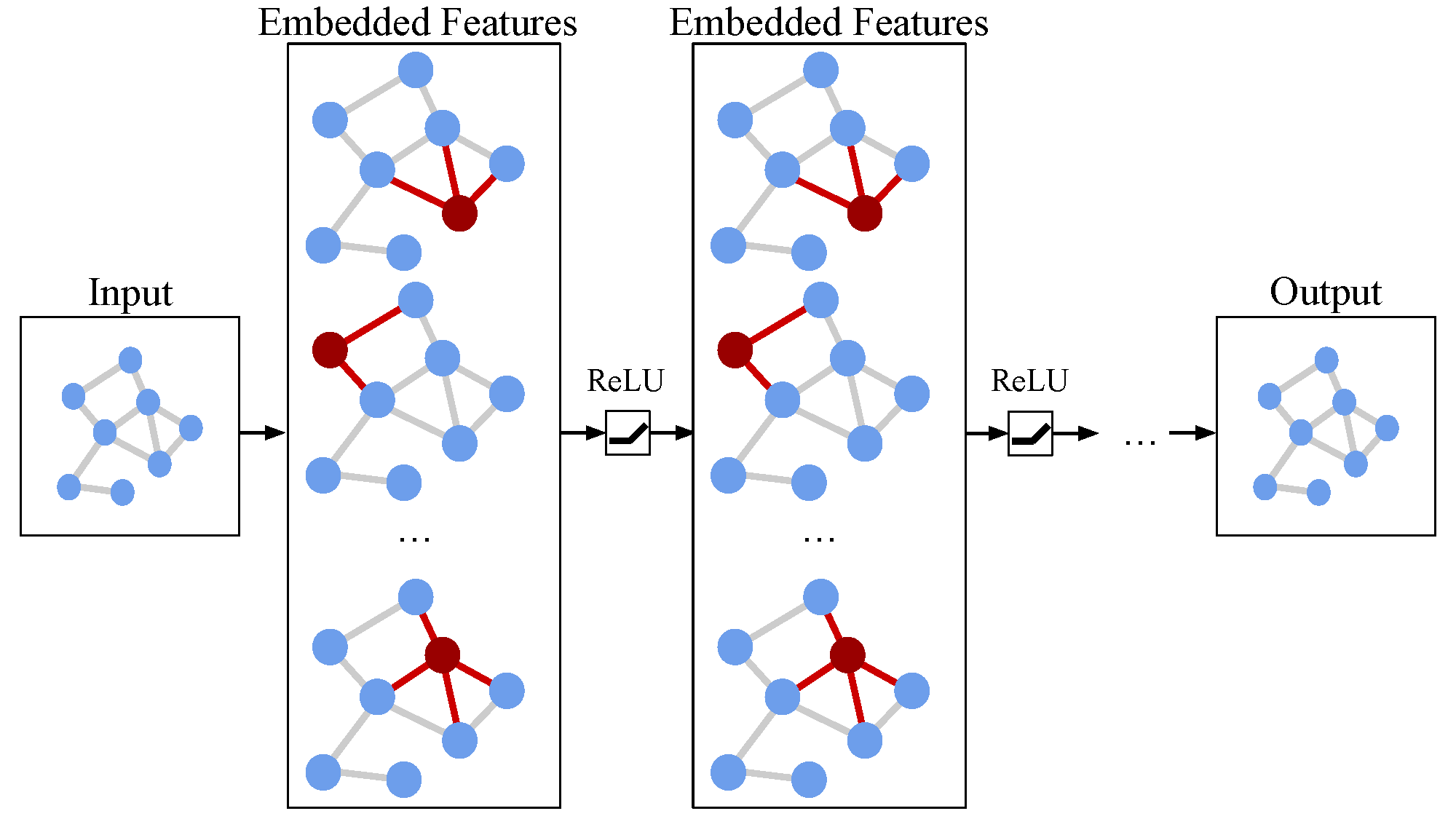
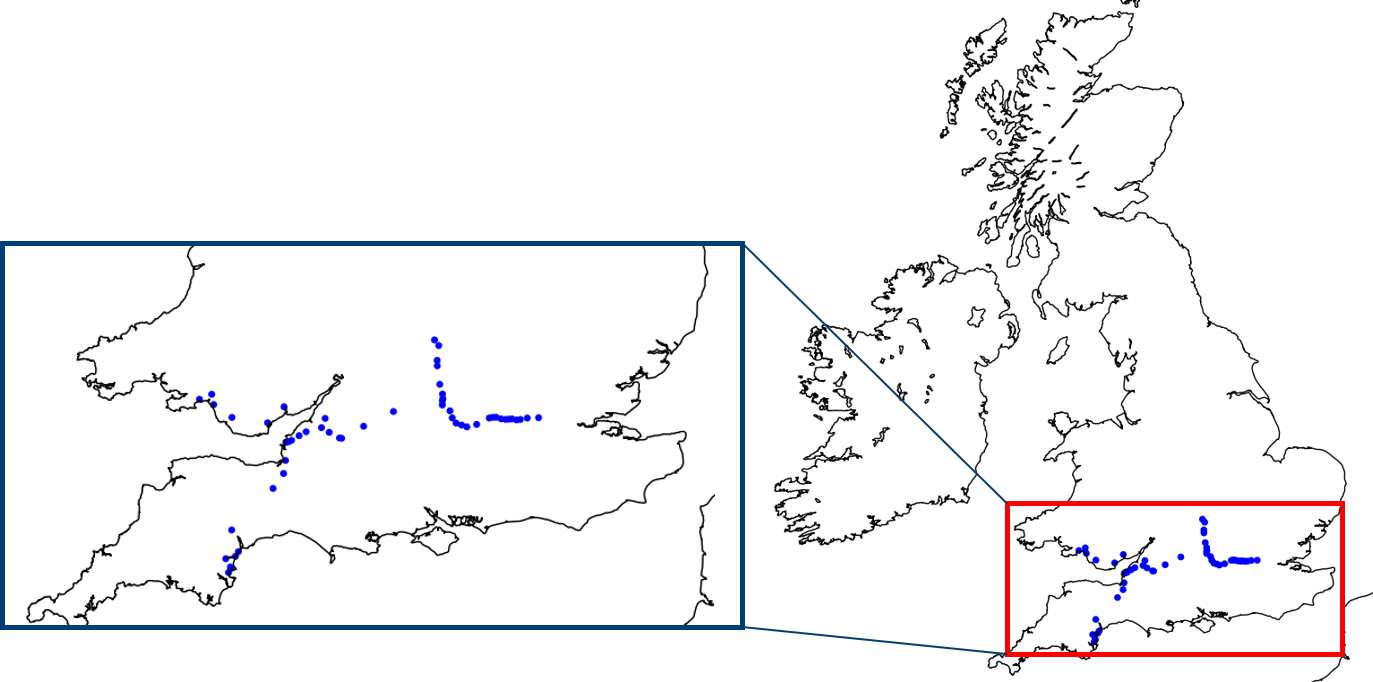
This work leverages spatio-temporal graph neural networks (STGNNs) to predict delays in railway networks. We find that STGNNs improve predication accuracy relative to linear and vanilla multi-layer perceptron models. See our ITSC paper presenting our results for more details.